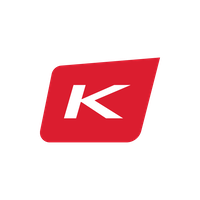
Attribute based forecast generation can be defined as using the common attributes of a particular group of products to more accurately forecast future demand at the individual SKU level. Forecasting is part science and part art, so the more we can move the analysis to the science side, the better we are able to develop a process driven forecasting model not based on an individual’s ‘gut’ feel. This is an important requirement when managing a large number of SKUs in a complex, competitive marketplace. The benefits of increased forecast accuracy include:
- Increased customer satisfaction due to reduced stock outs
- Reduced write-off and obsolescence costs due to unsold inventory
- Lower inventory carrying costs, as less buffer stock is required to cover missed forecasts
Significant benefits can be realized in a company’s top and bottom line results if a more accurate forecast generation process can be realized. One of the cardinal rules of forecasting is that a forecast becomes more inaccurate as it goes further out in time. Another is that a forecast becomes more inaccurate as the granularity is increased (ie. It is easier to get an accurate forecast at the business unit revenue level than it is to forecast the number of units of each SKU that make up the sales for that business unit). This second rule can be better addressed by focusing not an individual SKUs sales history or possible future trends, but by looking at the ‘group’ this SKU(s) fall into and analyzing its history and trends. The attributes shared by these SKUs constitute their forecast group. The advantage of grouping forecast SKUs are many:
- A lot of market research is available at a higher level in the marketplace (at the channel product category level versus an SKU level). Market share analysis must be done at this level
- Aggregating SKUs reduces the month to month demand fluctuations seen at the SKU level, allowing for better data analysis for trend forecasting (reduces the noise)
- Revenue forecasting generally occurs at the product group level or higher. Since revenue projections are the most common driver of forecast models, a better alignment between forecasts at the SKU level and revenue forecasts can be achieved if there is a clearly understood link between the two
- A better alignment of revenue and SKU forecasts allows the business to more clearly drive production (also known as the sales versus production forecast). A poor alignment between the two can lead to missed sales numbers, increased inventory carrying costs, or stock outs.
The breakdown of the revenue forecast into a realistic SKU level forecast can be complex, and can vary greatly among different business units and markets. While these different processes can lead to a more accurate forecast based on local market conditions, it makes it very difficult to get a true picture of the alignment between the global revenue forecast and the individual unit forecasts, as the methods to get from one to the other can vary greatly.
This leads us to the recognition for the need of a formal, structured process for generating SKU unit forecasts from revenue forecasts that can generate a better forecast for the business as a whole. In order to allow the process to accommodate the differences between markets and regions, it must be have the functionality to allow for market specific processes, while maintaining a common underlying process that can tie back to the revenue forecast.
The best way to achieve this goal is to use region and market independent product attributes, as they can ‘filter out’ the variations that can occur due to different business cultures. This also describes the best product attributes to select, as they should reinforce the underlying commonality of the SKUs being forecast. Market specific processes can then be applied to fine tune the forecast for a specific market or region.
In order to more accurately predict future demand at the SKU level, a tool is required can process large amounts of historical data and knowledge based assumptions quickly. This allows the analysts to perform what if simulations and determine the effects of various assumptions, as well as incorporate any trends that are evident in the marketplace.
This leads to another "must have" feature: the ability for the analyst to easily and quickly incorporate trend simulations into the numbers. By using aggregated attributes on multiple levels and presenting the numbers in a percentage of total view, the analyst can quickly modify the splits at each attribute level to reflect known assumptions or model future trends, while keeping the totals within the revenue guidelines (the sum of the percentages must always equal 100). As well, by modifying percentages versus actual numbers at the lower attribute level, the overall revenue forecast can be kept consistent throughout the forecast process.
The revised percentage splits can then be applied to the revenue numbers to break them down into a SKU level forecast that ties back to the revenue in an understandable way. In summary, it is desirable to have a close correlation between the revenue forecast and the SKU level forecast. The best way to get there is to use product attributes, historical patterns, and the analyst’s expert knowledge to break the revenue forecast down to an accurate SKU level forecast.
A tool that can support an underlying, formalized process with enough flexibility to allow for regional and market differences will deliver the optimum solution. This tool must be intuitive to use and allow the analyst to quickly generate a valid forecast that supports the consensus revenue projections.